Journal.ean.edu.co
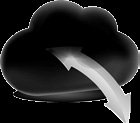
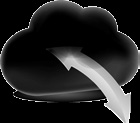
Indicators of ADHD symptoms
FECHA DE RECEPCIÓN: 23 de junio
in virtual learning context using
FECHA DE APROBACIÓN: 17 de julio
Pp. 22-37
machine learning technics
Laura Patricia Mancera Valetts*
Indicadores de síntomas ADHD en
Silvia Margarita Baldiris Navarro**
el contexto de aprendizaje virtual,
Viviana Betancur Chicué***
utilizando técnicas de aprendizaje
Indicateurs de symptômes de
Troubles et Déficits d'Attention
causés par l'Hyperactivité (TDAH)
en contexte d'apprentissage
virtuel à l'aide de techniques
Indicadores dos sintomas TDAH
na aprendizagem virtual
Contexto usando técnicas de
* Máster en informática industrial, automática y de sistemas. e-Learning-Universitat de Girona;
Ingeniera de sistemas, informática y administración -Universidad Autónoma de Bucaramanga;
Docente investigadora de la Universidad Manuela Beltrán
**Postdoctorado University of Athabasca Research Methods in Education;
Doctorado en Tecnologías de la Información-Universitat de Girona ;
Maestría/Magister Universitat De Girona
Máster en Informática Industrial y Automática
Ingenieria de Sistemas-Universidad Industrial de Santander - UIS. Docente Universidad
Manuela Beltrán
***Maestría en EducaciónUniversidad Pedagógica Nacional;
Licenciatura en Educación Física y desplegamiento- Universidad Pedagógica Nacional;
Docente Universidad Manuela Beltrán.
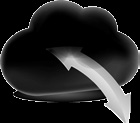
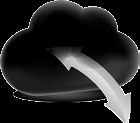
Rev. esc.adm.neg. No. 79
Bogotá, Pp.22-37
RESUMEN
Este artículo presenta un proceso de modelado de usuario, específicamente un modelado
de estudiante, en un ambiente virtual de aprendizaje, que permite inferir la presencia o no de síntomas del Déficit de Atención e Hiperactividad (TDAH). El modelo de usuario es
Déficit de atención e
construido teniendo en cuenta tres características del estudiante: Conducta de comportamiento
hiperactividad, sistemas
(BC), Rendimiento de funciones ejecutivas (EFP), y estado emocional (ES). Para inferir
hipermedia adaptativos,
si un estudiante puede tener un perfil asintomático de TDAH, se usa un grupo de reglas de
plataformas virtuales de
clasificación que usan los resultados obtenidos en cada característica como datos de entrada
aprendizaje, modelo de
para su funcionamiento. Basados en las pruebas del modelo propuesto, se obtiene un grupo
usuario, reglas de clasificación,
de entrenamiento que es usado para preparar un algoritmo de aprendizaje automático, el
aprendizaje automático.
cual podrá realizar y mejorar la tarea de crear el perfil para cada estudiante de acuerdo a si presenta o no síntomas del TDAH o problemas de atención. Esto, puede ser el primer paso para ofrecer recursos de aprendizajes adaptados a las necesidades educativas de estudiantes que presenten este trastorno.
This paper presents a user model for students performing virtual learning processes. This model is used to infer the presence of Attention Deficit Hyperactivity Disorder (ADHD)
Attention deficit
indicators in a student. The user model is built considering three user characteristics, which
hyperactivity disorder,
can be also used as variables in different contexts. These variables are: behavioral conduct
adaptive hypermedia system,
(BC), executive functions performance (EFP), and emotional state (ES). For inferring the
virtual learning platform, user
ADHD symptomatic profile of a student and his/her emotional alterations, these features
modeling, machine learning
are used as input in a set of classification rules. Based on the testing of the proposed model,
technics, classification rules.
training examples are obtained. These examples are used to prepare a classification machine learning algorithm for performing, and improving, the task of profiling a student. The proposed user model can provide the first step to adapt learning resources in e-learning platforms to people with attention problems, specifically, young-adult students with ADHD.
RESUMÉÉ
Cet article présente un modèle d'utilisateur type chez les étudiants inscrits en mode
d'apprentissage virtuels. Ce modèle est utilisé pour prévenir chez les élèves la présence de
Troubles et Déficits d'Attention
Troubles et Déficits d'Attention causés par l'Hyperactivité (TDAH). Le modèle est construit
causés par l'Hyperactivité,
pour prendre en compte trois caractéristiques de l'utilisateur qui peuvent aussi être utilisées comme variables dans différents contextes. Ces variables sont : la conduite et comportement
Système Hypermédias
(CC), la performance (P) et l'état émotionnel (EE). Pour déduire le profil symptomatique
Adaptatifs, Plate-forme
de TDAH d'un étudiant et de ses altérations émotionnelles, ces fonctionnalités sont utilisées
Virtuelle d'Apprentissage,
comme données dans un ensemble de règles de classification. Ces exemples sont utilisés
Modélisation de l'Utilisateur,
pour préparer un algorithme d'apprentissage automatique de classification et permettent
Règles de Classification
d'améliorer l'analyse du profil d'un élève. Le modèle d'utilisateur type peut offrir la première étape pour l'adaptation des ressources d'apprentissage aux plates-formes d'enseignement à distance pour des personnes atteintes de troubles de l'attention, en particulier chez les jeunes étudiants atteints de TDAH.
Este artigo apresenta um modelo de usuário para o desempenho dos estudantes nos processos
de aprendizagem virtual. Este modelo é usado para inferir a presença de indicadores de Déficit de Atenção com Hiperatividade (TDAH) em um estudante. O modelo de usuários é
Desordem de Déficit de Atenção
construído considerando três características dos usuários, as quais podem também ser usadas
com Hiperatividade, Sistema
como variáveis em diferentes contextos. Essas variáveis são: conduta de comportamento,
de Hipermídia Adaptativa,
desempenho de funções executivas e estado emocional. Para inferir o perfil sintomático
Plataforma de Aprendizagem
do TDAH de um estudante e seus/suas alterações emocionais, estas características são
virtual, Modelagem do
usadas como entrada em um modelo de regras de classificação. Baseado no teste do modelo
Usuário, Aprendizado de
proposto, exemplos de treinamento são obtidos. Esses exemplos são usados para preparar
Técnicas de Máquina, Regras
um algoritmo de desempenho da preparação da classificação, e melhorar, a habilidade de
de Classificação.
perfilar um estudante. O modelo do usuário proposto pode prover o primeiro passo para adaptar os recursos de aprendizagem nas plataformas de aprendizagem virtual para pessoas com problemas de atenção, especificamente, estudantes que são adultos-jovens com TDAH.
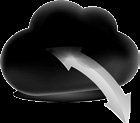
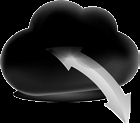
Indicators of ADHD symptoms in virtual learning context using machine learning technics
Bogotá, Pp.22-37
In the context of e-Learning, many efforts the learning process according to users' features.
have been conducted to address the A variety of studies have been developed due to individual user's needs to provide adaptive
this merger (Baldiris, Santos, Barrera et al, 2008;
learning processes. To accomplish this purpose,
Baldiris, Santos, Huerva et al, 2008; Jurado
the integration of Adaptive Hypermedia Systems
et al, 2008). This integration, which aims to
(AHSs) with Virtual Learning (VL), also known provide personalized learning and management as Adaptive Virtual Learning Environment was processes, is a comprehensive field of research. proposed (Tiarnaigh, 2005; Colan et al, 2002).
This is because within this field, there is a
AHSs are systems with the ability to adapt diversity of users' characteristics and needs, and themselves accordingly for each individual user,
a diversity of technologies that VLPs support.
providing a more personalized and customizable
Within the large variety of users that utilize VLPs,
course to every user. The distinctive elements of
people with disabilities, disorders or illnesses are
an AHS are: a) a user model, which represents
included. Technology might help these people to
information about a particular user, essential for
achieve quality learning.
adaptive systems in order to provide adaptation effects; and b) an adaptation process, which is
This work is centered on young-adults
the mechanism that decides how the system with Attention Deficit Hyperactivity Disorder should be adapted, according to the features (ADHD), especially in a university context, who represented in the user model (Brusilvsky & are included in a long life learning process. The Millan, 2007). This paper is focused on the user
reasons for focusing on this group of people are:
a) during the last few years, the number of people diagnosed with ADHD has been growing (Kessler
On the other hand, Virtual Learning et al, 2006), b) the research conducted on ADHD
Platforms (VLPs) are applications that use Internet
is mostly focused on children and adolescents,
as a way to publish and
forgetting adult population (Peninington &
provide tools for the Ozonoff, 1996; Kroes et al, 2001), c) several management of courses,
studies have demonstrated that individuals with
contents and services. this disorder might have difficulties and letdowns, These applications offer
such as school and job failures (Faraone et al,
a good alternative to the
2000), and d) several studies have reported that
classroom-based environment since they could most students with deficits such as those that be customized by an administrator in order to
compose the ADHD, who take online courses,
suit the needs of specific groups or class of drop them in few days because they find the learners (Colan et al, 2002). However, in many
courses hard to follow (Grabinger, 2010).
VLPs, the content that might be customized is non-adaptive (i.e., the content is the same for
Based on the above mentioned, our
all the students).
work is focused on providing adult people with ADHD an alternative to perform their education
The benefit of integrating VLPs and AHSs
with quality learning processes. In this paper,
lies in combining the strengths of both systems;
we present a user modeling approach to infer
VLPs provide administration and support if a specific student may be suffering from facilities, while AHSs provide a personalization of
ADHD symptoms. A user model is then created
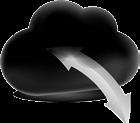
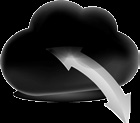
Laura Patricia Mancera Valetts/ Silvia Margarita Baldiris Navarro/ Viviana Betancur Chicué
considering three features: behavioral conduct,
This paper is structured as follows: section
cognitive performance and emotional state. The
2 explains some of the impairments related to the
proposed user model can provide the first step
ADHD that we considered in this work. Section
to adapt learning resources in LMSs to people
3 provides some outlines of our proposal and
with attention problems, specifically, student-
describes the user model. Section 4 describes
young-adults with ADHD.
the machine learning process to support future classification of people with ADHD. Finally, section 5 presents some concluding remarks and future work.
2. ATTENTION DEFICIT HYPERACTIVITY DISORDER
ADHD is a neurobiologically and neuropsycho-
with ADHD show a primary dysfunction in the
logical y heterogeneous disorder characterized by
recognition of emotional stimuli. Furthermore,
inattention, hyperactivity and impulsivity (NIMH,
these people present an important disability to
2012). In neuropsychological studies performed
control their emotions, specifically the negative
on people with ADHD, impairments in some ones such as aggressiveness, depression, sadness, of their cognitive functions, specifically, failures anger and frustration.
in executive functions (EF) were found (Toplak, Jain,
Considering both cognitive and emotional
& Tannock, 2005; Mulas et
deficiencies, a recent model, known as the Dual
al, 2006; Etchepareborda, Pathway Model, states that in ADHD, there are Paiva-Barón, & Abad, 2009;
separate pathways for cognitive deficits and
Barkley, 1997; Brown, 2002).
motivational deficits. An individual with ADHD
‘Executive function' has been conceptualized as
presents impairments in either one of these
a term that comprises interrelated higher-order
pathways, or both (Sonuga-Barke, 2003).
cognitive processes responsible for goal-directed and contextually appropriate behavior (Alvarez &
However, cognitive and/or motivational
deficits are not exclusive of ADHD and, at the present time, to evaluate the ADHD in adults is a
On the other hand, research on ADHD complex process. In general, to diagnose a person
has mainly focused on cognitive aspects, leaving
with ADHD requires performing an interview
in the background the study of the affective based on diagnostic criteria proposed by interna-deficiencies of the disorder. In this context, a tional experts grouped in the Diagnostic and variety of studies have stated that these affective
Statistical Manual of Mental Disorders -DSM-
deficiencies are also important for the adequate
IV (APA, 2000) or the International Statistical
diagnosis and treatment of the ADHD (Barkley,
Classification of Diseases and Related Health
1997; Nicolau, 2002; Reimherr et al, 2005; Problems (ICD-10) of the World Health Sonuga-Barke, 2003). Specifically, these studies
Organization (WHO) [22], executing retrospective
indicate that children, young people and adults
diagnosis of childhood symptoms, discarding
Indicators of ADHD symptoms in virtual learning context using machine learning technics
Bogotá, Pp.22-37
other illnesses or disorders, performing cognitive
behavior symptoms, (b) a key question that
tests, interviewing the evaluated person and
aims to discard other disorders, (c) a multimedia
its family, analyzing patient's behavior, and
version of cognitive tests to obtain deficits in
including, if necessary, more complex studies such
cognitive areas related to executive functions,
as Magneto-encephalography and Functional
and (d) emotion recognition techniques to
Magnetic Resonance.
recognize users' emotions in real-time. The user modeling process will support a future
In this work, the following processes are
solution for improving the quality of learning
considered to infer if a user may have ADHD
of people with ADHD. In this work, we are not
symptoms: (a) a self-assessment rating scale
intending to present a method for detecting
to characterize and to quantify relevant ADHD
ADHD in a medical context.
3. USER MODEL FOR QUALITY LEARNING PROCESSES
OF STUDENTS WITH ADHD
The characteristics that are considered in asked to perform a computer question-based
order to infer if a specific student could
test and some computer cognitive tasks. The
suffer from ADHD symptoms are obtained
tests are used to characterize and to quantify
by an evaluation of users' behavioral conduct,
relevant ADHD behaviors in a student. The
executive functions performance and emotional
computer cognitive tasks are used to evaluate
the students' executive functions performance. While the user interacts with the system, a
The general process to obtain information
mechanism of facial expression recognition
from the students in each of these characteristics
is executed to extract information about the
can be described as follows: the students are
emotions expressed by the user (Figure 1).
Figure 1. General process of our proposal.
Source. By the authors.
Laura Patricia Mancera Valetts/ Silvia Margarita Baldiris Navarro/ Viviana Betancur Chicué
As it is shown, we have considered positive answers are estimated to suggest the
the implementation of a set of external web presence of symptoms consistent with ADHD in applications to evaluate each characteristic adults. To mitigate false-positive results, a key considered in the students' profile. In this question that aims to discard disorders different manner, these tools can be connected with than ADHD has been included. The result of the a variety of VLPs. The way we used to embed
evaluation for the behavior conduct is either
the set of web applications with the ATutor positive or negative. e-learning platform was the inline frame. Besides, we used the php5-uuid and php5-curl functions to generate a standard identifier also known as universally unique identifier (UUID) to
3.2 Executive Functions
enable Web applications and ATutor to uniquely
Performance
identify information without significant central coordination; however, some elements in the To extract information about the executive VLPs need to be adjusted, or incorporated, to
function performance of a user, computer-based
complete the user modeling process, these are
neuropsychological tests/tasks are frequently
(a) the creation of a data structure, where the
used. The tests used in this research allow
information related to the profile is stored, and
to evaluate the following areas of the EF: (a)
(b) the definition of classification rules, from Sustained Attention (SA), which refers to the which the user' profile is built.
ability to maintain a stable performance level over a period of time, (b) Mental Flexibility (MF),
An explanation of each of the characteristics
which is the capacity of shifting the focus of
considered for the user profile and their usage to
attention and moving between tasks having
infer if a student may be suffering from ADHD
different cognitive requirements, (c) Working
symptoms is presented below.
Memory (WM), which is the capacity to store, monitor and manage information, and (d) Verbal Learning (VL), which refers to the capacity to obtain, hold and remember words. We evaluate
3.1 Behavioral Conduct
these areas, which are deficient in people with ADHD (Marchetta, 2007). In this work, we apply
In order to characterize and to quantify user the Sustained Attention Task (SAT) (Wilkins, behaviors that may be relevant to ADHD Shallice, & McCarthy, 1987), to evaluate the symptoms, the short version of the Adult ADHD
sustained attention area; the Concept Shifting
Self-Report Scale – v1.1 (ASRS v1.1) is used Test (CST) (Van der Elst et al, 2006), to check (Daigre et al, 2009). This scale is proposed by
mental flexibility; and the Rey Auditory Verbal
the WHO and validated for Spanish speakers. Learning Test (RAVLT) (Rey, 1958) to evaluate The scale is consistent with the evaluation the last two areas, working memory and verbal criteria of DSM-IV (APA, 2000). The test consists
learning. Even though there are a number of
of six items and takes less than five minutes to
implementations of these cognitive tests for
be completed. The evaluation scale for each computer, we implemented our own tools. This item has five possible answers: never, rarely, is because most of these implementations are sometimes, often and very often. In the first given as licensed software.
three items, the options from sometimes to very often are considered as negative, and in the last
Generally in these kinds of test, statistics
three items, the options from often to very often
are used to define the performance of a
are recognized as the positive ones. Four o more
patient. We compare the patients' results with
Indicators of ADHD symptoms in virtual learning context using machine learning technics
Bogotá, Pp.22-37
the normalized data, according to age and
As it is shown, the possible results of the
education level of people without cognitive executive functions performance are: very high, problems on each of the three tests (Rey, 1958;
high, medium, low, and very low. The ranges for
Rey, Feldman, Rivas et al, 1999). Considering the final classification are obtained considering that we have different evaluation scales for the
the limits of the possible results (e.g. two very
tests, we compare the patients' results on each
low results [2x1] and one low [2] should produce
test to the mean (μ) of its normalized data in
a final very low [4], but two low results [2x2]
terms of its standard deviation (σ). Based on and one very low [1] should produce a final low the guidance of Ferran Viñas Poch, PhD, and result [5]. The rest of the ranges are constructed Beatriz Caparrós Caparrós, Psychologists with in the same manner).
experience on ADHD and associate professors from the Girona University, we classify the students' results using the parameters defined immediately (Table 1).
3.3 Emotions shown by the user
Table 1. Patient results per each test.
The emotions shown by a particular user allow supporting the detection of ADHD symptoms.
The set of emotions that we are interested in
capturing are: sadness, anger, disgust, fear,
happiness and a neutral state. These emotions
Between μ - 2 σ and μ- σ Low
will be obtained from the users' facial expressions
Between μ - σ and μ + σ
through a real-time emotion recognition system.
Between μ + σ and μ + 2σ High
The expressions are captured using images
taken with a webcam while the user is working
Source. By the authors.
For the final executive functions perfor-
The standard process to build a real-time
mance result, a number from 1 to 5 is assigned
emotion recognition system consists of four
for the possible results on each of the three tests.
steps: (1) image acquisition, which is the process
The final result is obtained by adding the values
of capturing the users' image; (2) face detection,
acquired in these tests. The scale for the final
in which using the captured image, the face is
executive functions performance classification is
detected; (3) feature extraction, which is the
shown below (Table 2).
process of extracting information associated with the activation of different muscles of the
Table 2. Range for final executive functions
face; and (4) emotion classification, which uses
performance classification.
the information acquired in the previous step to
decide the most probable emotion of the user in
a specific moment.
For the first step, a web application
that captures a video from the webcam of
the student and splits the video into frames is
utilized. The Adobe Flash Builder is used for this
implementation. Machine learning methods will
Source. By the authors.
be used for steps 2, 3 and 4, considering that good results have been obtained applying these
Laura Patricia Mancera Valetts/ Silvia Margarita Baldiris Navarro/ Viviana Betancur Chicué
techniques for classifying expressions of basic 3.4 Classification rules
emotions (Littlewort, Bartlett, Fasel et al, 2004; Michel & El Kaliouby, 2003).
The abovementioned characteristics can be used in contexts different than ADHD. A set
In the second and third steps, the Machine
of classification rules must be defined then,
Perception Toolbox (MPT), which contains to use the results of the evaluation of these
open source libraries for real-time perception characteristics for determining if a user presents
primitives, will be used. Using the MPT, face ADHD symptoms and/or emotional alterations.
detection, eye detection, blink detection and color tracking can be performed (Littlewort,
In order to define the classification rules,
Bartlett, Chenu et al, 2004). This toolbox is the psychologists in this work suggested
based on a system developed by Viola and Jones
that (a) behavioral conduct should present
(2001), consisting on a cascade of classifiers higher significance than executive functions
trained by boosting techniques. The MPT performance; (b) the emotional state should
includes enhancements to the process proposed
be only used to define if the student presents
by the Viola and Jones face detector (e.g., emotional alterations. Using these rules, the
employing the Gentleboost algorithm instead student might be classified into one of the
of the Adaboost, and enabling smart feature following four classes: (1) ADHD symptomatic-
search and a novel cascade training procedure,
free, (2) ADHD symptomatic without emotional
as in Viola & Jones, 2001).
alterations, (3) ADHD symptomatic with emotional alterations, and (4) ADHD symptomatic-free with
For the fourth step, a K-nearest neighbor
possible emotional alterations.
rule will be used in order to check how our system works. The Support Vector Machines method is
When the classes corresponding to No
planned to be used in future implementations.
ADHD symptoms (with or without emotional
This is because some studies have demonstrated
alterations) occur from a positive in the
the effectiveness of this algorithm in the emotion
behavioral conduct and a normal, high or very
classification task (Michel & El Kaliouby, 2003).
high executive functions performance, the student may present another disorder different
The emotion recognition system begins than ADHD or could be a false negative. In the
when the user authorizes the capture of the same manner, these situations may happen
images from his/her webcam. The complete when those classes of No ADHD symptoms
process to obtain the emotional state will be occur from a negative in the behavioral conduct
repeated constantly. Then, an analysis of the and a very low or low executive functions
different emotions shown by the student is performance.
performed. This analysis consists in determining the predominant emotion from the captured
The tree (Figure 2), shows the rules that
group, i.e. the emotion that repeats the most in
have been defined for the student classification.
the group. Since the system works in real-time, this predominant emotion can change in time.
Indicators of ADHD symptoms in virtual learning context using machine learning technics
Bogotá, Pp.22-37
Figure 2. Classification tree.
Normal (3). High (4)
Normal (3). High (4)
and Very high (5)
and Very high (5)
Source. By the authors.
4. SUPPORTING USER MODELING PROCESS THROUGH
MACHINE LEARNING TECHNIQUE
Since our overall proposal is to offer The first step refers to the acquisition of
adaptive learning resources to students the training and test sets of instances used to with ADHD, the user modeling process prepare the classification algorithm. Data for
is an important issue. This is because the user
these sets will be provided for the results of
model provides the approach to identify if a some test scenarios, where users are asking to specific student presents ADHD symptoms.
present the computer test submitted before in order to know the ADHD symptoms. Results
However, the presence of ADHD from other studies such as the one developed
symptoms might be a reason why students do
by Marchetta (2007), might be also used for
not finish the tasks used for the classification.
defining the training and test sets even if these
A machine learning classification task has results consider areas that are more cognitive been proposed then, to infer if a student who
than the ones used for this work.
did not finish these tasks might have ADHD symptoms. The automatic classification process
The second step of the process is to
has been divided into three steps: information
define the best algorithms for developing the
acquisition, classification algorithm selection, classification task. In this work, this refers to the and classification process validation.
best algorithm to classify a user in the adequate ADHD symptomatology. We will test some classification algorithms such as ID3 (Quinlan,
Laura Patricia Mancera Valetts/ Silvia Margarita Baldiris Navarro/ Viviana Betancur Chicué
1986), ID4.5 (Quinlan, 1993), and also some • The attributes and their values are: age (a mixed algorithms using boosting and bagging
discrete number among 18 to 60), education
processes. The design of the classification task is
level (EL) (between 7 and 12 years of studies,
determined as follows:
13 or more years of studies), BC (positive or negative), MF-CP (very low, low, medium,
• The object task: to classify a particular user
high and very high), WM/VL-CP (very low,
according to few input data in a specific
low, medium, high and very high), SA-CP
ADHD symptomatology characterization.
(very low, low, medium, high and very high)
• The possible values for the class are: (a) ADHD
bb and R-TE (happiness, sadness, anger,
symptomatic-free, (b) ADHD symptomatic
disgust, fear and neutral). The characteristics
without emotional alterations, (c) ADHD
in parentheses are the possible values for
symptomatic with emotional alterations, and
each of the attributes.
(d) ADHD symptomatic-free with possible emotional alterations.
The third step is the validation of the
classification process, measuring the precision of the task and other features.
Table 3. Shows some examples of instances that might be used for the training set.
Attribute
Frustrated No DHD
I: Instance, A: Age, E.L.: Education Level, B.C.: Behavioral Conduct, M.F.: Mental Flexibility, W.M.V.L: Working Memory and Verbal Learning, S.A.: Sustained Attention, E.S.: Emotional State, +: Positive B.C., -: Negative B.C., ADHD+: Possible ADHD symptoms, ADHD- : No ADHD symptoms.
Source. By the authors.
Instances 4 and 5 can be possible cases of disorders different than ADHD or false negatives (Table 3).
Indicators of ADHD symptoms in virtual learning context using machine learning technics
Bogotá, Pp.22-37
5. CONCLUSION AND FUTURE WORK
The overall goal behind this work is to We plan to test a variety of
provide quality learning processes to classification algorithms in order people with attention problems, specifically
to choose those that offer better
to adults with ADHD. This is because these accuracy. Furthermore, we also people may find the classical education hard plan to use another classification to follow, and might find in virtual learning an
algorithm in the context of the
alternative to perform their education. We have
automatic emotion recognition to improve this
built a user model that considers some deficient
characteristics in the ADHD, which can be used to infer if a specific student may have ADHD
Considering the findings referring to
symptoms. The work presented in this paper can
other neurological and psychiatric disorders that
be the first step to deliver personalized learning
may present the same deficiencies as ADHD
resources to students with the ADHD.
through this research, we plan to expand the range of users to which this development can
For improving the results, a machine be addressed. On one hand, this project can be
learning classification task is suggested instead
extended to other disorders by applying specific
of a manual classification task. Two main rating scales for each of them; on the other reasons for using machine learning classification
hand, the project can offer a generalized user
are when the classification task is large for model that considers cognitive and emotional manual performance, and when the value dysfunctions.
of a characteristic is absent for a student, the machine learning algorithm can still infer the
Although psychologists are supervising
classification for that particular student.
the performance on this work in order to build a system as realistic as possible, we are not
The expected result of applying machine
intending to present a method for detecting
learning techniques is that this automatically ADHD in a medical context. We would performed classification can be compared with
recommend a person to look for professional
those suggested by professionals on the ADHD.
advice in case this person feels this disorder is
This comparison will indicate how good the seriously affecting her/ his life. provided diagnosis is.
Laura Patricia Mancera Valetts/ Silvia Margarita Baldiris Navarro/ Viviana Betancur Chicué
Alvarez, J.A. & Emory, E. (2006). Executive function and the frontal lobes: A meta-analytic review.
Neuropsychology Review, 16(1), 17 – 42.
American Psychiatric Association. (2000). Diagnostic and Statistical Manual of Mental Disorders,
Fourth Edition - Text Revision (DSMIV-TR).
Baldiris, S.M., Santos, O.C., Barrera, C., Boticario, J.G., Velez, J., & Fabregat, R. (2008). Integration
of Educational Specifications and Standards to Support Adaptive Learning Scenarios in ADAPTAPlan. International Journal of Computer & Applications, 5(1), 88—107.
Baldiris, S.M., Santos, O.C., Huerva, D., Fabregat, R., Boticario, J.G. (2008). Multidimensional
Adaptations for Open Learning Management Systems. WI-IAT ‘08 Proceedings of the 2008 IEEE/WIC/ACM International Conference on Web Intelligence and Intelligent Agent Technology, 3, 352-356. Washington, DC: IEEE Computer Society.
Barkley, R.A. (1997). Behavioral inhibition, sustained attention, and executive functions: constructing
a unifying theory of ADHD. Psychol Bull 121, 65-94.
Brown, T.E. (2002). DSM-IV: ADHD and executive function impairments. Advanced Studies in
Medicine, 2, 910-914.
Brusilvsky, P. & Millan, E. (2007). User Models for Adaptive Hypemedia and Adaptive Educational
Systems. The Adaptive Web, 4321(1), 3-53. Berlin, Germany: Springer-Verlag Berlin.
Colan, O., Wade, V., Gargan, M., & Hockemeyer, C. (2002). An architecture for integrating adaptive
hypermedia services with open learning environments. Proceedings of World Conference on Educational Multimedia, Hypermedia and Telecommunications. Denver, CO: EDMEDIA.
Daigre, C., Ramos-Quiroga, J.A., Valero, S., Bosch, R., Roncero, C., Gonzalvo, B., Nogueira, M., &
Casas M. (2009). Cuestionario autoinformado de cribado de TDAH ASRS-v1.1 en adultos en tratamiento por trastornos por uso de sustancias. Actas Esp Psiquiatr, 37(6), 299-305.
Etchepareborda, M.C., Paiva-Barón, H., & Abad, L. (2009).The advantages of neuropsychological
examination batteries in attention deficit hyperactivity disorder. Rev Neurol, 48 (2), S89-93.
Faraone, S., Biederman, J., Spencer, T., Wilens, T., Seidman, L., Mick, E., et al. (2000). Attention-
Deficit/Hyperactivity Disorder in adults: An Overview. Biological Psychiatry, 48, 9—20.
Grabinger, S. (2010). A framework for supporting postsecondary learners with Psychiatric Disabilities
in Online environments. Electronic Journal of e-learning, 8(2), 101-110.
Indicators of ADHD symptoms in virtual learning context using machine learning technics
Bogotá, Pp.22-37
Jurado, F., Santos, O., Redondo, M., Boticario, J., & Ortega, M. (2008). Providing Dynamic Instructional
Adaptation in Programming Learning. Proceedings of the 3rd International workshop on Hybrid Artificial Intelligence Systems, 329-336. Berlin, Germany: Springer-Verlag Berlin.
Kessler, R.C., Adler, L., Barkley, R., Biederman, J., Conners, C.K., Demler, O., Faraone, S.V., et al.
(2006). The prevalence and correlates of adult ADHD in the United States: results from the National Comorbidity Survey Replication. American Journal of Psychiatry, 163(4), 716-23.
Kroes, M., Kalff, A.C., Kessels, A.G.H., Steyaert, J., Feron, F., Van Someren, A.J.W.M., et al. (2001).
Child psychiatric diagnoses in a population of Dutch school-children aged 6 to 8 years. Journal of the American Academy of Child and Adolescence Psychiatry, 40(12), 1401—1409.
Littlewort, G., Bartlett, M.S., Chenu, J., Fasel, I., Kanda, T., Ishiguro, H., & Movellan, J.R. (2004).
Towards social robots: Automatic evaluation of human-robot interaction by face detection and expression classi cation. Advances in neural information processing systems, 16, 1563-1570. Cambridge, MA: MIT Press.
Littlewort, G., Bartlett, M.S., Fasel, I., Chenu, J., & Movellan, J. R. (2004). Analysis of Machine
Learning Methods for real-time recognition of facial expressions from video. Computer Vision and Pattern Recognition. MPLab TR 2003.05.
Marchetta, N.D. (2007). Cognitive processes in adults with ADHD. Maastricht, The Netherlands:
Neuropsych Publishers, Maastricht University.
Michel, P. & El Kaliouby, R. (2003). Real Time Facial Expression Recognition in Video using Support
Vector Machines. In Proceedings of the 5th international conference on Multimodal interfaces, 258-264.
Mulas, F., Etchepareborda, M.C., Abad-Mas, L., Díaz-Lucero, A., Hernández, S., De la Osa, A.,
Pascuale, M.J., & Ruiz-Andrés, R. (2006). Neuropsychological disorders in teenagers with attention deficit hyperactivity disorder. Rev Neurol, 43(1), S71-S81.
National Institute of Mental Health. (2012). Attention Deficit Hyperactivity Disorder. Bethesda, MD:
NIMH Science Writing, Press and Dissemination Branch. Retrieved from: http://www.nimh.nih.
gov/health/publications/attention-deficit-hyperactivity-disorder/complete-index.shtml.
Nicolau i Palou, R. (2002). TDAH: el control de las emociones. Fundación ADANA Problemas asociados
al TDAH. IIª Jornada.
Peninington, B.F. & Ozonoff, S. (1996). Executive Functions and Developmental Psychopathology.
Journal of Child Psychology and Psychiatry, 37(1), 51—87.
Quinlan, J. R. (1986). Induction of decision trees. Machine learning, 1(1), 81-106.
Quinlan, J.R. (1993). C4.5: Programs for Machine Learning. San Mateo, CA: Morgan Kaufmann
Publishers, Inc.
Laura Patricia Mancera Valetts/ Silvia Margarita Baldiris Navarro/ Viviana Betancur Chicué
Reimherr, F.W., Marchant, B.K., Strong, R.E., Hedges, D.W., Alder, L., Spencer, T.J., et al. (2005).
Emotional dysregualation in adults with ADHD and response to atomoxetine. Biol Psychiatry, 58, 125-131.
Rey, A. (1958). L'Examen Clinique en Psychologie. Paris: Press Universitaire de France.
Rey, G.J., Feldman, E., Rivas-Vazquez, R., Levin, B.E., Benton, A. (1999). Neuropsychological test
development and normative data on Hispanics. Archives of Clinical Neuropsychology, 14, 593-601.
Sonuga-Barke, E.J. (2003). The dual pathway model of AD/HD: an elaboration of neuro-developmental
characteristics. Neuroscience & Biobehavioral Reviews, 27(7), 593-604.
Tiarnaigh, M. (2005). Adaptive Moodle: An integration of Moodle (Modular Object-Oriented Dynamic
Learning Environment) with an AHS (Adaptive Hypermedia System). PhD Thesis, University of Dublin.
Toplak, M.E., Jain, U., & Tannock, R. (2005). Executive and motivational processes in adolescents
with Attention-Deficit-Hyperactivity Disorder (ADHD). BioMed Central, Behavioral and Brain Functions. doi: 10.1186/1744-9081-1-8.
Van der Elst, W., Van Boxtel, M.P.J., Van Breukelen, G., & Jolles, J. (2006). The Concept Shifting Test:
Adult Normative Data. Psychological Assessment, 18(4), 424–432.
Viola, P. & Jones, M. (2001). Robust real-time object detection. International Journal of Computer
Vision, 4, 51-52.
Wilkins, A.J., Shallice, T., & McCarthy, R. (1987). Frontal lesions and sustained attention.
Neuropsychologia, 25, 359–65.
Source: http://journal.ean.edu.co/index.php/Revista/article/viewFile/1265/1226
Volume 2, Number 1, June 2009 ISSN 1995-6681 Pages 1 -6 Jordan Journal of Earth and Environmental Sciences Diurnal and Seasonal Variation of Air Pollution at Al-Hashimeya Sana'a Abed El-Raoof Odat * Department of Earth Science and Environment, Faculty of Natural Resources and Environment,Hashemite University, Jordan Abstract
Neurofeedback – How Attention Takes Flight Pierre Walther and Stephan Ellinger Goethe University Frankfurt, Julius-Maximillians-University Würzburg (GERMANY) Attention Deficit Disorder (ADD) alone or in combination with Hyperactivity (ADHD) is one of the most common disorders in childhood and adolescence and even persists into adulthood. Children with ADHD show a higher amount of slow brain waves and a decreased amount of faster brain waves compared to children without ADHD (Barry et al., 2003). The basic idea of neurofeedback is to transfer the unconscious process of brain wave function into a conscious process by reporting it to the patient. The Brainfeeders project aims to evaluate the possibilities for integrating neurofeedback in a school setting. The primary goal of the study is to replicate results found in clinical trials without any additional human resources. We would like to evaluate how well a training programme like this fits in school settings and if results are comparable to clinical studies. We are interested in forming a transnational working group, integrating researchers who are working on similar projects or who are interested in working on Brainfeeders in their countries.