Egitim.erciyes.edu.tr
Mini-Reviews in Medicinal Chemistry, 2005, 5, 479-487
Human Acetylcholinesterase Inhibitors: Electronic-Topological and Neural
Network Approaches to the Structure-Activity Relationships Study
F. Kandemirli*, 1, M. Saraçoglu2 and V. Kovalishyn3
1Department of Chemistry, Kocaeli University, 41000, Kocaeli, Turkey
2Department of Chemistry, Erciyes University, 38039, Kayseri, Turkey
3Biomedical Department, Institute of Bioorganic Petroleum Chemistry, 253660, Ukraine
Abstract: The Electronic-Topological (ETM) and Neural Network methods were applied to the study of the
"structure–acetylcholinesterase (AChE) inhibitor activity" relationships for a series of physostigmine and N-
benzylpiperidine derivatives. Molecular fragments specific for active compounds and breaks of activity were
calculated for human AChE by applying the ETM and Neural Network methods. Requirements necessary for a
compound to be active were formulated; they are the result of detailed analysis of all compounds under study. A
comparative study of the activity features found for human AChE was performed.
Keywords: Human AChE Inhibitors, Electronic Topological Method, Neural Network Method.
determined or calculated) that relate to physico-chemical properties of the compounds. The QSARs of
Alzheimer's disease (AD), the most common cause of
AChE inhibitors were reviewed recently [8-16];
dementia in the elderly, is a chronic, slowly progressiveneurodegenerative disorder with characteristic deterioration of
Electronic-topological method (ETM), which is a
intellectual capacity in various domains: learning and
structural approach designed for the investigation of
memory, language abilities, reading and writing, praxis,
structure-property relationships. In "structure-AChE
interaction with the environment. One of the few undisputed
inhibitor activity" [17] relationship studies had been
evidences in the neuropathology of the AD is the loss of
performed for three series of N-benzylpiperidine
cholinergic neurons occurring in different areas of the central
derivatives by using the ETM. The results of the
nervous system, mainly the cerebral cortex and the
study show how the use of the ETM makes it
hippocampus [1-5].
possible to bypass the incompatibility problem as tothe experimental data.
One promising therapeutic strategy for activating central
cholinergic functions has been the use of inhibitors of
The present study that uses the ETM and Neural
acetylcholinesterase (AChE). This enzyme is responsible for
Network methods as well, aims at finding new AChE
the metabolic hydrolysis of acetylcholine. Tacrine,
inhibitors that can be useful against AD.
donepezil, and rivastigmine are acetylcholinesteraseinhibitors that increase the levels of acetylcholine at thesynapse by blocking the breakdown of the neurotransmitter
MATERIALS AND METHODS
Data Sets
Previous computational studies can be divided into three
Compounds under study (73 molecules in total [18-22]
are shown in Table
1. Their common structural skeletons are
given in (Fig.
1). that shows how compounds under study
Approaches used to model ligand-receptor interaction
belong to different structural classes. Skeletons
A, B and
F
through docking (molecular dynamics); these have
represent various derivatives of 4-(3-benzisoxazolylethyl)-N-
been applied to only small series [7];
benzylpiperidine. Molecular skeletons
C, D, and
E represent
Quantitative structure-activity relationship (QSAR)
modifications performed on the structure of the natural
studies that use either Comparative Molecular field
analysis (CoMFA) or conventional 2D QSARmethods. Mainly, this methodology aims at the
Table
1 reports calculated and experimental log 1/IC50,
development of simple mathematical models that
measured on human erythrocyte AChE of 73 compounds.
correlate changes in biological activity with variations
Molecules under study were classified as active compounds
in the structure of molecules. These variations are
(31 molecules with log 1/IC50 ≥ 7.24), low-active ones (13
accounted for by parameters (experimentally
molecules with 7.22 ≤ log 1/IC50 ≤ 6.81) and inactivecompounds (29 molecules with log 1/IC50 < 6.80).
To identify activity features (or pharmacophores), the
*Address correspondence to this author at the Department of Chemistry,
ETM-calculations were carried out twice: first, low-active
Kocaeli University. Kocaeli, Turkey; Tel: +90 262 528 57 61; Fax: +90
compounds were considered as belonging to the active class,
262+3215968; E-mail:
[email protected]
and then as belonging to the inactive class.
2005 Bentham Science Publishers Ltd.
480 Mini-Reviews in Medicinal Chemistry, 2005, Vol. 5, No. 5
Kandemirli et al.
In Vitro Inhibition of Human AChE by Physostigmine and N-Benzylpiperidine Derivatives and Prognostigation of
Activity
log 1/IC50
log 1/IC50
2,6-(C2H5)24 -CH3Ph
Mini-Reviews in Medicinal Chemistry, 2005, Vol. 5, No. 5 481
Fig. (1). Common molecular skeletons of the compounds under study.
ET Method Description
follows a common scheme for the pattern recognition, but ithas also some peculiarities that stem from complexity of the
The ETM can be considered as one of structure-based
"structure-property" problem. A pattern recognition-based
approaches [23-26]. As all structural methods, it needs a
application consists of the following stages. The first stage is
language for the compounds structure description (LCSD).
known as
data transforming, when input data must be
Because three-dimensional (3D) graphs are taken as models
prepared in a predefined format. In the ETM-scheme, this
for real molecules, mathematical structures used in the ETM
stage serves for an ETMC formation for every molecule Si.
as LCSD are commonly used in QSAR matrices (one matrix= one compound). However, the nature of their elements is
The second stage,
pre-processing (known also as
feature
different. Instead of some global chemical properties
selection) is to be done, aiming at the definition of common
(lipophilicity, solubility) used in QSAR methods, the ETM
molecular fragments (i.e. congruent subgraphs SjA, j∈J; "A"
uses quantum-chemical, or electronic characteristics and data
means "responsible for the given activity"). They are
taken from conformational analysis. Conformational analysis
searched for in
all structures Si, i∈I. A straightforward
and quantum chemistry calculations were carried out by
solution is to search them by comparing, one by one,
means of molecular mechanics method (MMP2) and semi-
molecular structures Si with a
template S0 (an active
empirical quantum chemistry method (AM1), respectively.
compound, if features of activity are searched). Algorithmsapplied at this stage are named
self-
learning procedures. The
Diagonal elements of the matrices called Electronic-
corresponding algorithm of the ETM needs a number of
Topological Matrices of Contiguity (ETMC, for short)
initial parameters of the ETM algorithm. They are:
reflect one or more atomic properties (represented by aseparate value or a vector of characteristics). Off-diagonal
A threshold of activity, which allows the separation of
elements characterise bonds between pairs of atoms, if they
all compounds into corresponding groups;
exist, or distances, otherwise. (Usually, only the upper
A template molecule for the comparison;
triangle of the matrix is used in calculations because of thesymmetry of bonds.) Values of the bond properties can be
Values ∆1-3 that are used to fix a definite level of
also represented by one or more values. However, only one
flexibility of molecules;
value is used in calculation for simplicity. If there are more
A desired value of a criterion
CA(SjA) (probability of
a
than one properties for atoms and bonds, the ETM
fragment
SjA
presence
in the set {SkA} of molecular
calculations can be repeated for every separate property. The
formal description of the ETM can be found in [17].
The estimation of the probability for a SjA, is calculated
Computational part of the ETM is a sequence of the
by the following formula proved in structural methods:
CA (SjA) = (
LA+1)/(
LA +
LNA +2)
Conformational analysis
Where
LA,
LNA are numbers of compounds from the
{SkA} and {SkNA} sets, respectively, which contain the SjA
fragment. If the fragments found satisfy the criterion
CA andare informative enough, from the point of view of the
Processing ETMCs (the search of the structural
researcher, the procedure stops. Otherwise, it is repeated with
features responsible for activity/inactivity by
different initial settings.
comparing a template active/inactive compound withthe rest of compounds).
Next important stage in both indirect methods and some
structural ones is an
examination procedure. In the case of
The last two steps represent the essential part of the
the ETM application, however, the found fragments can be
ETM. The core of the ETM-software (see Fig.
2) mainly
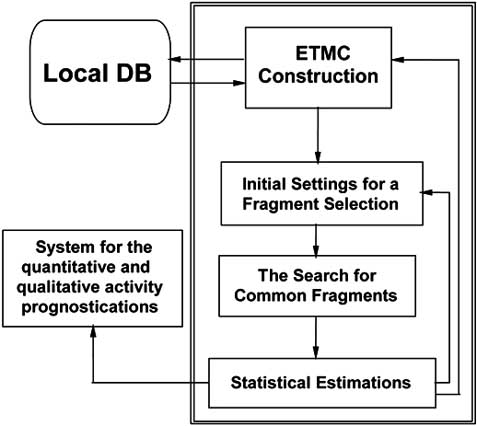
482 Mini-Reviews in Medicinal Chemistry, 2005, Vol. 5, No. 5
Kandemirli et al.
Fig. (2). Common scheme of ETM.
used immediately, if they satisfy some conditions
When used together, both types of features represent valuable
superimposed by the researcher. At the same time, the
information for the design of new compounds with the
examination procedure is applied when the researcher wants
desired activity A.
to validate the stability of the fragments selected. This isdone by the "leave-one-alone" technique, when allcompounds from the set {Sk
NEURAL NETWORK METHOD
A} are tried as templates for
matrices' comparison. In contrast to many other methods in
Artificial Neural Networks (ANNs) is a group of methods
the ETM, this task is fulfilled by the same procedure as the
that are increasingly being used in drug design to study
one used at the previous step. The features validation is
QSAR [27, 28]. This method is able to elucidate structure-
obligatory, when a quantitative (functional) model is to be
activity relationships and take into account any non-linear
developed on the basis of the fragments found (as usually is
character of these relationships. Thus, this method can be of
the case of indirect methods).
significant interest in 3D QSAR studies.
The concluding step is the development of a set of
For the analysis of the data we have used one of the most
decision rules for the activity (A) prediction. In the ETM,
well-known neural networks - the feed forward neural
this set is represented by a set of molecular substructures
networks (FFNNs) trained with the back propagation
(represented by submatrices of ETMCs, or by ETSA, for
algorithm [29, 30]. The architecture of the ANNS was
short) and some numerical data that are important for
consisted of three-layers with five neurons in one hidden
recognising new molecular structures possessing the activity
layer. One single output node was used to code activities of
A. When having such set, the given property P prediction
AChE inhibitors. The bias neuron was presented on the
consists in the search of congruent subgraphs in all
input and on the hidden layer. At least M=200 independent
abovementioned structures Si. Again, this procedure follows
FFNN were trained to analyse each set of variables. The
the same steps as the procedures for features selection and
predicted values of each analysed case were averaged over all
validation, but its initial settings ∆1-3 and CP are those
M network predictions and the means were used to calculate
calculated at the first step, and templates are exactly the
statistical coefficients with targets. The other details of the
features selected.
algorithm can be found elsewhere [31, 32].
When successfully found, a feature SjA elucidates core
The avoidance of overfitting/overtraining has been shown
mechanisms of the receptor-ligand interactions. But a similar
to be an important factor for the improvement of predictive
study can be carried out when the user wishes to find ‘breaks
ability and correct selection of variables in the feed forward
of activity', which indirectly characterise sterically
neural networks [31]. The Early Stopping over Ensemble
inaccessible and/or electronically forbidden regions of
(ESE) technique was used in the current study to accomplish
receptors. They are fragments that are common to all
this. A detailed description of ESE can be found in [31, 32].
compounds from {SlNA, for all l} and cannot be found in the
In brief, each analysed artificial neural network ensemble
compounds from {SkA, for all k}. (It is noteworthy that for a
(ANNE) was composed of M=200 networks. The values
break of activity SjNA the criterion CNA looks similar.)
calculated for analysed cases were averaged over all M neural
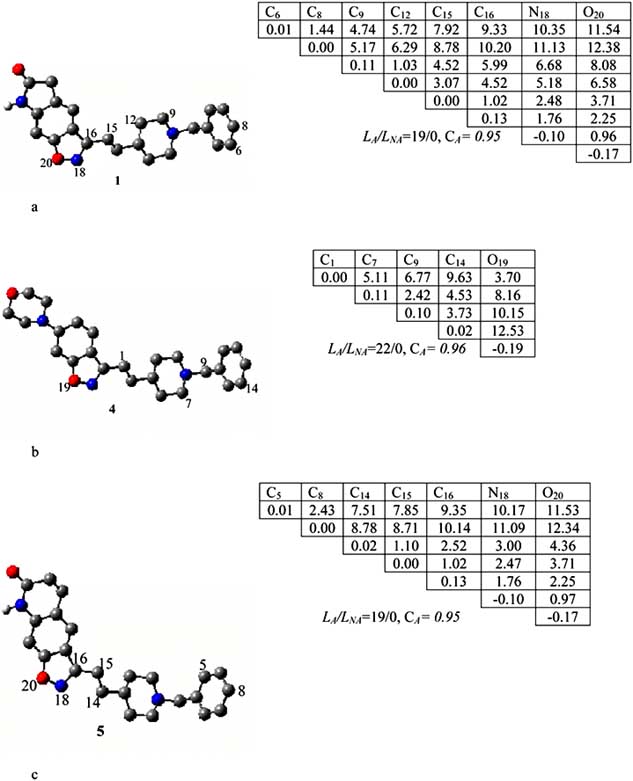
Mini-Reviews in Medicinal Chemistry, 2005, Vol. 5, No. 5 483
Fig. (3). The P1(a), P2(b) and P3(c) pharmacophores found relative to active molecules 1, 4 and 5 respectively.
networks, and their means were used for computing
last improvement of root–mean-square error in the early
statistical coefficients with targets. We used a subdivision of
stopping point. The root–mean-square error E was computed
the initial training set into two equal learning/validation
as a criterion of network learning to determine the stop
subsets. The first set was used to train the neural network
points of a training procedure. The quality of the model was
while the second one was used to monitor the training
tested by the leave-one-out cross-validation q2 value defined
process measured by root mean square error. An early
stopping point determined as a best fit of a network to the
q2 = (SD-press)/SD;
validation set was used to stop the neural network learning.
Thus, statistical parameters calculated at the early stopping
Introduced by Cramer et al. [33]. Here SD represents the
point were used. The training was terminated by limiting
variance of a target value relative to its mean and 'press' is
the network run to 10, 000 epochs (total number of epochs)
the average squared errors of predicted values obtained from
or after 2, 000 epochs (local number of epochs) following the
leave-one-out (LOO) procedure.
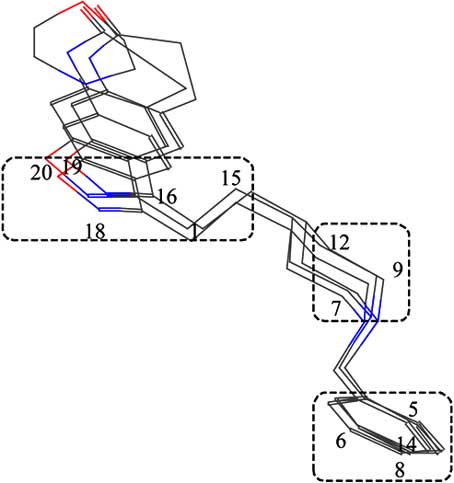
484 Mini-Reviews in Medicinal Chemistry, 2005, Vol. 5, No. 5
Kandemirli et al.
The LOO cross-validation procedure was used to
The pharmacophore was found in 19 of 31 active
supervise the predictive performance of ANN.
compounds, and it was not found in inactive compounds at
all. Thus, the probability CA of its realisation in the active
It has been shown that pruning algorithms [34, 35] may
class is about 0.95. As seen from the pharmacophore's
be used to optimise the number of input parameters for
structure, it consists of the C6, C8 atoms of the phenyl, and
ANNs learning and to select the most significant ones.
C9, C12, C15, C16, N18, O20 atoms.
These algorithms operate in a manner similar to step-wisemultiple regression analysis and exclude on each step one
Pharmacophore P2 was calculated relative to the template
input parameter that was estimated to be non-significant.
molecule 4 in a similar way as for P1 (see Fig. 3b). The P2
The pruning algorithms were used in the current study to
was found in 22 active compounds and it was not found in
determine significant parameters of input data points of the
inactive compounds at all (correspondingly, the probability
analysed molecules as described in references [34, 35].
of its appearance is estimated as 0.96). The P2 includes five
atoms in total, as seen from (Fig. 3b).
RESULTS AND DISCUSSION
Pharmacophore P3 was calculated by taking compound 5
as the template for comparison. The P3 includes seven
According to the common scheme of the ETM,
atoms, which are carbon atoms C5, C8, of the phenyl cycle
conformational analysis and quantum chemistry calculations
and two carbons, C14, C15, C16, N18, O20. They belong to
were carried out for all compounds in the series under study.
different parts of the template molecule, and, as seen from
As the result of the conformational analysis, conformational
(Fig. 3c), they represent the most important two parts of all
structures with global minimum of their energies are to be
active molecules.
In Figure 4, the superimposition of three template
compounds that correspond to the calculated pharmacophores
Pharmacophores and Anti-Pharmacophores Calculation
P1 – P3 is shown. In 3D space three separate regions can beindicated for each active molecule, where atoms of the three
Electronic and steric parameters responsible for the
pharmacophores can be found.
activity form a matrix, which is a submatrix of thecorresponding template ETMC. As it was already said, such
These regions are shown in the (Fig. 4) by dotted lines.
submatrix is called the electron-topological submatrix of
One of them is formed by the atoms belonging to the phenyl
activity (ETSA). So, the activity feature (or pharmacophore)
ring, while the other two are formed by the atoms that
P1 was calculated by taking molecule 1 as template
represent the heterocyclic rings. It is quite possible that the
compound (∆1= ± 0.05, ∆2= ± 0.10). The P1 is shown in
atoms from these regions play an important role in the
(Fig. 3a) along with its ETSAP1.
Fig. (4). Template compounds 1, 4 and 5 alignment by superposing the P1, P2 and P3 features.
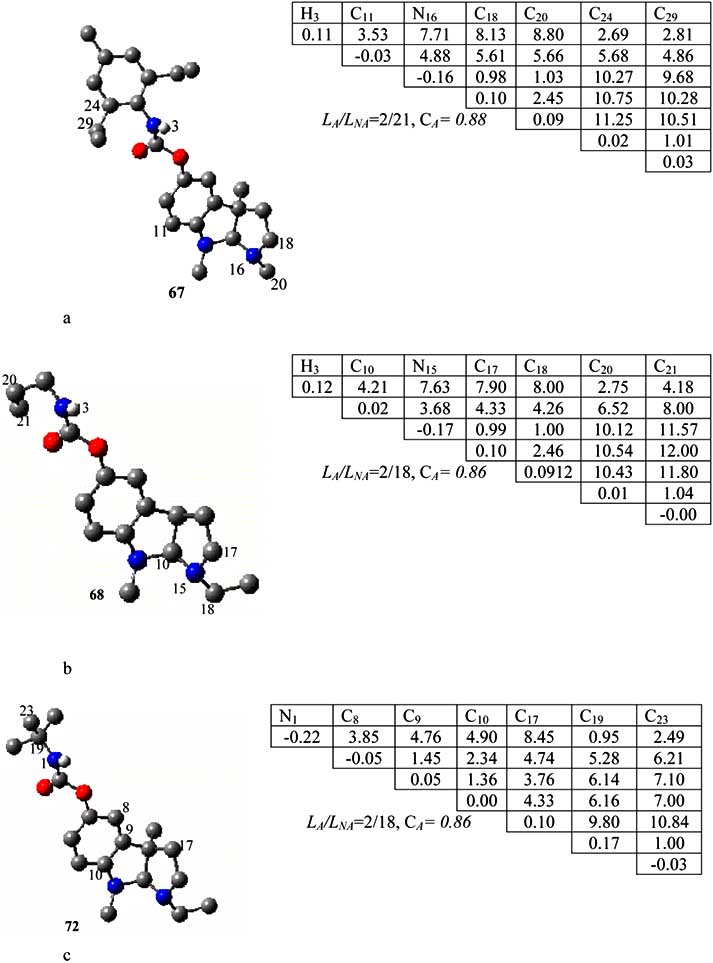
Mini-Reviews in Medicinal Chemistry, 2005, Vol. 5, No. 5 485
Fig. (5). The AP1(a), AP2(b) and AP3(c) anti-pharmacophore found relative to inactive molecules 67, 68 and 72 respectively.
When building a system for the activity prediction, those
interatomic 3D distances for the atoms can be seen from its
fragments of molecule are also to be taken into account,
ETSCAP1. 3D distances used at place of some off-diagonal
which are capable of deactivating an active structure (‘breaks
elements in the ETSAAP1 are close enough. AP1 feature was
of activity', or anti-pharmacophores). An anti-pharmacophore
found in 21of 29 inactive compounds. It was found 2 in
AP1 was found from template compound 67 (see Fig. 5a).
active compounds. So, the probability of its realisation
AP1 contains seven atoms belonging to different parts of the
(CNA) in this class of compounds is about 0.88.
template molecule 67. These atoms are C3, C11 C16, C18,
Anti-pharmacophore AP2 was calculated from the
C20, C24 and C29. Peculiarities of electronic-topological
template compound 68. The atoms C
parameters such as atomic charges, bond multiplicities and
3, C10, C15, C17, C18,
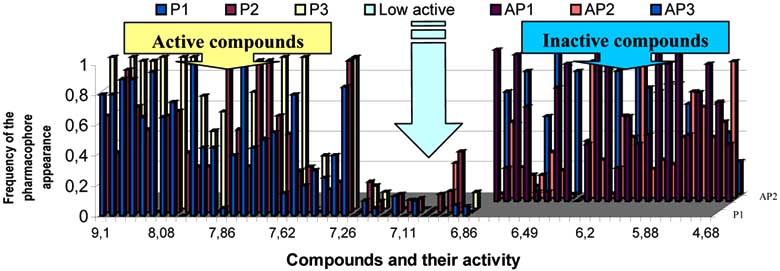
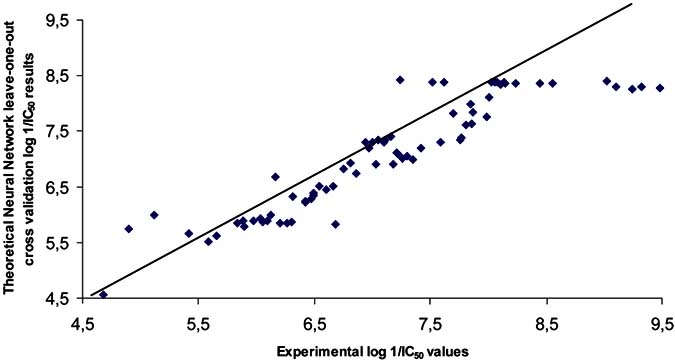
486 Mini-Reviews in Medicinal Chemistry, 2005, Vol. 5, No. 5
Kandemirli et al.
Fig. (6). Frequency of the fragments' occurrence in the compounds from the studied series for pharmacophores P1 ÷ P3 and for anti-
pharmacophores AP1 ÷ AP3.
C20, and C21 depicted in (Fig. 5b) were found incapable of
The ability of the aforementioned system to divide
hydrogen bonds formation with the receptor. The AP2 was
compounds of the training set into classes of
found in 18 of 29 inactive compounds and 2 active
activity/inactivity is illustrated in (Fig. 6) by frequencies of
compounds. Thus, the probability of its realisation was
the fragments occurrence in the compounds of the training
calculated as 0.86.
set. The frequencies are shown in dependence with the levelof AChE inhibition activity of the compounds in view.
From the compound 73 taken as the template, the anti-
pharmacophore AP3 was calculated. The activity feature was
As seen from the graph in the (Fig. 6), in the class of
found in 18 inactive compounds and in two of active
active compounds there is a group of high- active
compounds. As seen from (Fig. 5c), AP3 includes 7 atoms.
compounds and another group of compounds of moderate
The probability of its realisation in inactive compounds is
activity. The value of log 1/IC50 7, 22-6.81 serves as a
equal to 0.86. Molecules become inactive ones H replaces
boundary between the two groups.
methyl group in the R2 position to the D skeleton (see
molecules 46, 48, 54, 56, 58, 64, 68 in Table 1).
Neural Network Studies
A para-substituted phenyl decreases the inhibiting
activity of the compounds with skeleton C (compare
15 pharmacophores and 15 anti-pharmacophores
compounds 16, 43, and also compounds 20, 60). Elongation
descriptors were used as parameters for the analysis with
of the substituents attached to nitrogen atoms (compare
ANNs. The performance of neural networks was evaluated by
molecules 28, 31, 38 and 44) causes the increase of
LOO statistical coefficients calculated at early stopping point
inhibitory activity. The lengths of the chains of groups
for the training data set. The high cross-validation value q2 =
attached to molecules 44, 38, 28, 31 grow respectively.
0.78±0.01 confirms the validity of the model for predicting
Activity increases up to certain chain length (compounds 44,
activity of AChE inhibitors. At the second stage of analyses
38, 28) and then decreases with the further growth of the
we decided to examine, if all 30 descriptors attributes are
chain length (27, 31). The same situation was observed in
relevant for the prediction of activity AChE inhibitors.
the series of molecules 68, 64, 46 and 54 (Skeleton D).
Application of pruning methods allowed to select only nine
Fig. (7). Neural network leave-one-out cross validation log 1/IC50 results.
Mini-Reviews in Medicinal Chemistry, 2005, Vol. 5, No. 5 487
most relevant parameters (P1-P4, P11, P12, AP1-AP4,
Yamamoto, Y.; Ishihara, Y.; Kuntz, I.D. J. Med. Chem., 1994, 37,
AP6, AP10) responsible for AChE inhibitor activities. The
Rampa, A.; Bisi, A.; Valenti, P.; Recanatini, M.; Cavalli, A.;
calculated result shows that the cross-validation value q2=
Andrisano, V.; Cavrini, V.; Fin, L.; Buriani, A.; Giusti, P. J. Med.
0.81±0.01 as illustrated in (Fig. 7), a strong linear
Chem., 1998, 41, 3976.
dependency was obtained between the corresponding
Fukutu, T.R.; Metcalf, R.L. J. Agric. Food Chem., 1956, 4, 930.
predicted and experimental values of the AChE inhibitor
Hansch, C. Accounts Chem. Res., 1993, 26, 147.
Hansch, C.; Leo, A. Exploring QSAR. Fundamentals and
Applications in Chemistry and Biology. American Chem. Society.,
1995, Washington. DC, pp. 169.
This result confirms our hypothesis that pharmacophore
Hansch, C. Materials and Pharmaceuticals, ACS Symposium
and anti-pharmacophore parameters obtained from ETM
Series 589, American Chem. Society., 1995, Washigton. DC, pp.
method can be used for coding each molecule and building
Gupta, S.P. Chem. Rev., 1987, 87, 1183.
Magee, P.S. Chemicals Affecting Insects and Mites, in J.G.
Topliss (Ed.), Quantitative Structure Activity Relationships of
Drugs, New York, 1983.
Recanatini, M.; Cavalli, A.; Hansch, C. Chem.-Biol. Interactions,
Early research aimed at the development of quantitative
1997, 105, 199.
models for the structure-activity relationships in several
Tong, W.; Collantes, E.R.; Chen, Y.; Welsh, W.J. J. Med. Chem.,
1996, 39, 380.
series of AChE inhibitors have shown the importance of
Dimoglo, A.S.; Shvets, N.M.; Tetko, I.V.; Livingstone, D.J. Quant.
physical-chemical properties involved in the inhibitory
Struct.-Act. Relat., 2001, 20, 31.
activity [15]. As it follows from them, hydrophobicity,
Alisi, M.A.; Brufani, M.; Filicamo, L.; Gostoli, G.; Licandro, E.;
electronic and steric factors play a primary role in the
Cesta, M.C.; Lappa, S.; Marchesini, D.; Pagella, P. Bioorg. Med.
equations found as the result of that work. However the
Chem. Lett., 1995, 5, 2077.
Brzostowska, M.; He, X.; Greig, N.H.; Rapoport, S.I.; Brossi, A. J.
resulting equations have low values of correlation coefficients
Med. Chem. Res., 1992, 2, 238.
and are hardly appropriate for inhibitory activity
Chen, Y.L.; Nielsen, J.; Hedberg, K.; Dunaiskis, A.; Jones, S.;
Russo, L.; Johnson, J.; Ives, J.; Liston, D. J. Med. Chem., 1992, 35,
1429.
Systematic study was carried out by the ETM
Villalobos, A.; Blake, J.F.; Biggers, C.K.; Butler, T.W.; Chapin,
application in a series of compounds that are capable of
D.S.; Chen, Y.L.; Ives, J.L.; Jones, S.B.; Liston, D.R.; Nagel, A.A.;
demonstrating AChE inhibitory activity. Data obtained from
Nason, D.M.; Nielsen, J.A.; Shalaby, I.A.; Frost, W. J. Med.
Chem., 1994, 37, 2721.
conformation and quantum chemistry calculations were used
Villalobos, A.; Butler, T.W.; Chapin, D.S.; Chen, Y.L.; DeMattos,
to form electron-topological matrices. These matrices were
S.B.; Ives, J.L.; Liston, D.R.; Nagel, A.A.; Nason, D.M.; Nielsen,
effectively used to search for pharmacophores and anti-
J.A.; Ramires, A.D.; Shalaby, I.A.; Frost, W. J. Med. Chem., 1995,
pharmacophores. In Table 1 the results of the theoretical
38, 2802.
prognosis of the human AChE inhibitory activity are given.
Dimoglo, A.S. Khimiko-pharm. Zhurnal, 1985, (Rus) 4, 438.
Shvets, N.M. Comp. Sci. J. Moldova, 1993, 1, 101.
As follows from the Table, the system of three
Shvets, N.M. Comp. Sci. J. Moldova, 1997, 3, 301.
pharmacophores and three anti-pharmacophores effectively
Dimoglo, A.S.; Vlad, P.F.; Shvets, N.M.; Koltsa, M.N. New J.
separates compounds of the teaching set into groups of active
Chem., 2001, 25, 283.
and inactive compounds. Low-active molecules are badly
Kovesdi, I.; Dominguez-Rodrigue, M.F.; Orfi, L.; Naray-Szabo,
G.; Varro, A.; Papp, J.G.; Matyus, P. Med. Res. Rev., 1999, 19,
responsive to the activity prognostication because they form
a buffer zone consisting of compounds that can include both
Manallack, D.T.; Livingstone, D.J. Eur. J. Med. Chem., 1999, 34,
pharmacophores and anti-pharmacophores. The system is
supposed to be applied to screening and design of new active
Rumelhart, D.E.; Hinton, G.E.; Williams, R.J. Learning internal
compounds possessing skeletons similar to those used in the
representations by error propagation. In Parallel DistributedProcessing: Explorations in the Microstructure of Cognition,
present study.
Rumelhart, D.E.; McClelland, J.L.; Eds. M.A., The MIT Press,
Cambridge, 1986.
Zupan, J.; Gasteiger, J. Neural Networks for Chemistry and Drug
Design: An Introduction, 2 nd edition, VCH: Weinheim, 1999.
Tetko, I.V.; Livingstone, D.J.; Luik, A.I. J. Chem. Inf. Comput.
The authors thank Dr. N. Shvets for her assistance in
Sci., 1995, 35, 826.
carrying out the study.
Tetko, I.V.; Villa, A.E.P. Neural Networks, 1997, 10, 1361.
This coefficient was introduced as r2 in Cramer RD III, Patterson,
D.E.; Bunce, J.D. J. Am. Chem. Soc., 1988, 110, 5959-5967.
However, in order to avoid confusion with the analogous
conventional r2=R*R value the new designation q2 was
Davidsson, P.; Blennow, K.; Andreasen, N.; Eriksson, B.; Minthon,
recommended in Cramer III RD, De Priest, S.A.; Patterson, D.E.;
L.; Hesse, C. Neuroscience Letter, 2001, 300, 157.
Hecht, P. The Developing Practice of Comparative Field Analysis,
Snape, M.F.; Misra, A.; Murray, T.K.; De Souza, R.J.; Williams,
In: 3D QSAR in Drug Design: Theory Methods and Applications,
J.L.; Cross, A.J.; Green, A.R. Neuropharmacology, 1999, 38(1),
1993, H. Kubinyi Ed, ESCOM, The Netherlands, pp. 443-486.
Tetko, I.V.; Villa, A.E.P.; Livingstone, D.J. J. Chem. Inf. Comput.
Shadlen, M.F.; Larson, B. Postgraduate Med., 1999, 105(1), 109.
Sci., 1996, 36, 794.
Benzi, G.; Moretti, A. Eur. J. Pharm., 1998, 346(1), 1.
Kovalishyn, V.V.; Tetko, I.V.; Luik, A.I.; Kholodovych, V.V.;
Hasegawa, K. Neurobiology of Aging, 1998, 19, 107.
Villa, A.E.P.; Livingstone, D.J. J. Chem. Inf. Comput. Sci., 1998,
Obata, R.; Sunazuka T.; Otoguro, K.; Tomoda, H.; Harigaya, Y.;
Omura, S. Bioorg. and Med. Chem. Letters, 2000, 10, 1315.
Source: http://egitim.erciyes.edu.tr/~saracm/yayinlar/makaleler/yurtdisimakale/ydm-4(mrmc-1).pdf
Get inside to spot the BIG moves in SMALL COMPANIES SMALL "This year was our fiscal cliff. FY14 is when we realise all the hard work from our stategy. " Richard Pang, CEO ISS Group Welcome back to another year of Under the Radar. SHARE RESEARCH Tip 1. p2 mAynE pHARmA (myx)
les écoles partenaires ecole nationale de protection judiciaire de la jeunesse (eNPJJ) ecole nationale supérieure de la police (eNSPo) Centre national de la fonction publique territoriale (CNFPt) ecole des hautes études en santé publique (eHeSP) 10-12, Rue d'anjou avenue du Professeur Léon Bernard 75381 PaRIS Cédex 08 CS 7431235043 ReNNeS ecole nationale d'administration (eNa)